Lesson 1: Introduction to Weather Models
Objective: To provide a comprehensive understanding of how weather models are created, how they function, and how this knowledge enables users to interpret weather data effectively.
1.1 What is a Weather Model?
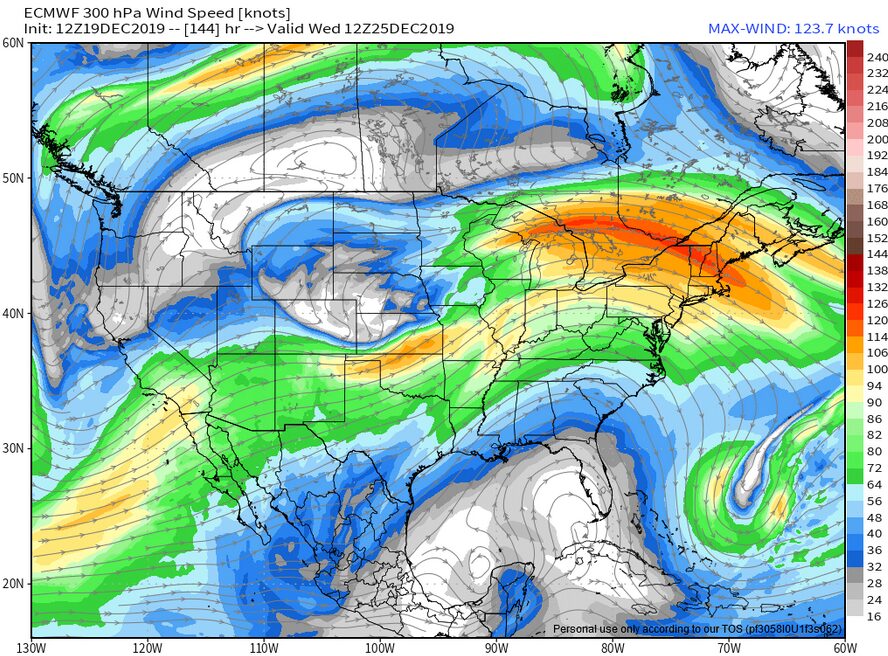
A weather model is essentially a virtual replica of the Earth’s atmosphere, constructed using advanced mathematics and physics. It employs complex algorithms and equations to simulate atmospheric processes based on fundamental physical laws like the conservation of mass, momentum, and energy. By inputting current weather observations into these models, meteorologists can project how the atmosphere will evolve over time, generating forecasts that range from hours to weeks ahead.
The foundation of weather modeling lies in the Navier-Stokes equations, which describe the motion of fluid substances such as air and water. These equations account for various forces acting on the atmosphere, including pressure gradients, Coriolis effects due to Earth’s rotation, friction, and external forces. Alongside these, thermodynamic equations consider heat exchanges resulting from radiation, conduction, convection, and phase changes of water.
Solving these equations analytically is impossible due to their nonlinearity and the chaotic nature of the atmosphere. Therefore, numerical methods are employed to approximate solutions at discrete points in space and time. This approach transforms the continuous equations into a set of solvable algebraic equations, allowing computers to simulate the atmosphere’s behavior.
Understanding what weather models are and how they function provides valuable insight into the complexity behind weather forecasting. This knowledge helps users appreciate the reliability of forecasts and the reasons behind uncertainties or variations among different predictions.
1.2 Data Collection
The accuracy of a weather model hinges on the quality and comprehensiveness of the data used to initialize it. Data collection is a massive, global effort involving a network of observations from the Earth’s surface to the upper atmosphere.
Surface Observations come from thousands of weather stations worldwide, both automated and manual. These stations measure variables such as temperature, humidity, wind speed and direction, atmospheric pressure, and precipitation. Observations are typically recorded hourly, providing a continuous stream of data that captures the ever-changing conditions at ground level.
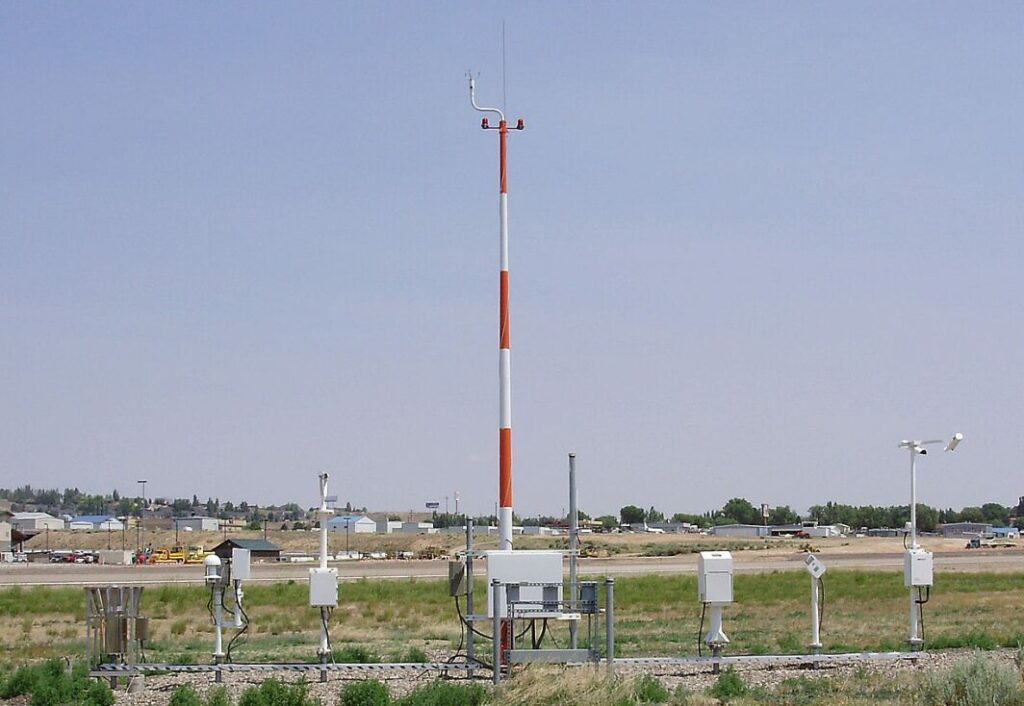
Automated airport weather station.
Upper-air Observations are obtained through weather balloons, or radiosondes, which are launched twice daily at 00Z and 12Z Coordinated Universal Time (UTC). These balloons ascend through the atmosphere, transmitting data on temperature, humidity, pressure, and wind at various altitudes. This vertical profiling is crucial for understanding the structure of the atmosphere and detecting features like temperature inversions or jet streams.
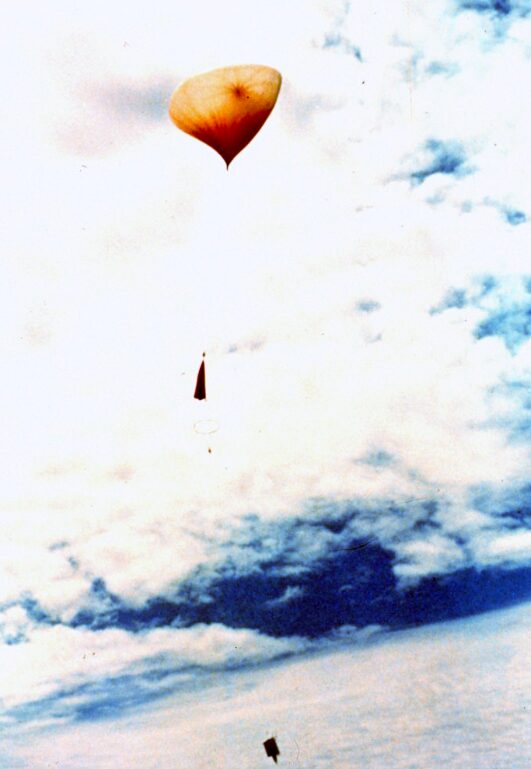
Weather balloon shortly after launch
Satellite Observations have revolutionized data collection by providing comprehensive coverage of the Earth’s atmosphere, including remote and oceanic regions where ground-based observations are sparse. Satellites come in two main types: geostationary satellites remain fixed over a point on the equator, offering continuous monitoring of weather systems, while polar-orbiting satellites circle the Earth from pole to pole, capturing global data over time. They measure variables such as cloud cover, sea surface temperatures, atmospheric moisture content, and more.
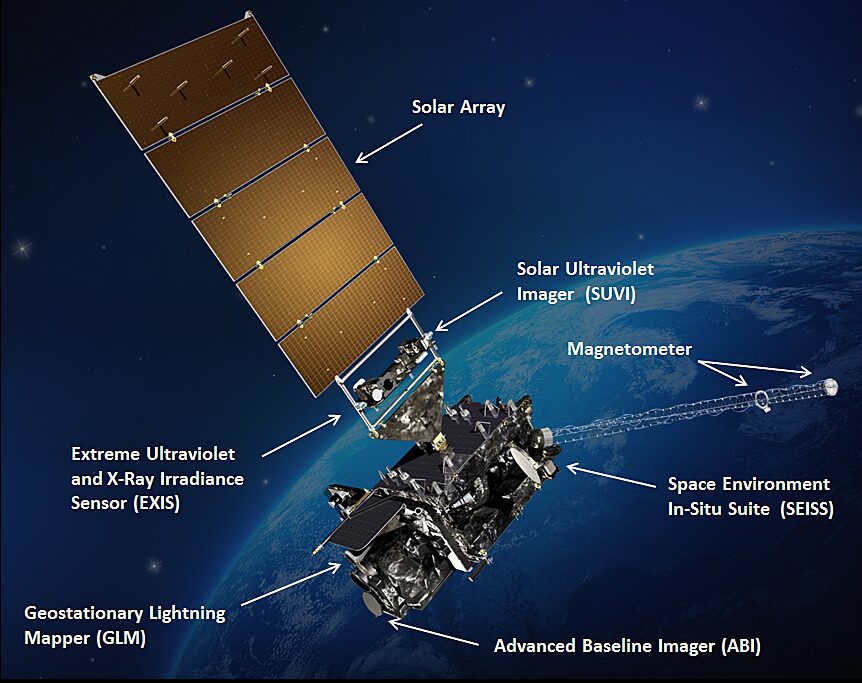
GOES-16, a United States weather satellite of the meteorological-satellite service
By NASA/NOAA – http://www.goes-r.gov/spacesegment/spacecraft.html (image link); see archived source website, Public Domain, https://commons.wikimedia.org/w/index.php?curid=58302419
Marine Observations from ships and buoys contribute valuable data over oceans, which cover about 70% of the Earth’s surface. These observations include sea surface temperatures, wave heights, wind speeds, and atmospheric pressure, filling gaps in areas where other data sources are limited.
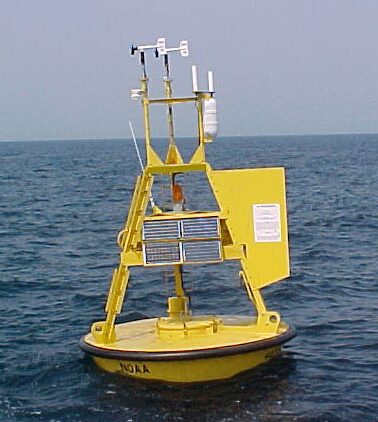
Weather buoy operated by the National Data Buoy Center
Aircraft Observations supplement upper-air data, especially over regions with sparse coverage. Many commercial aircraft are equipped with sensors that collect atmospheric data during flights, providing detailed information on temperature, wind, and sometimes humidity at various altitudes.
Aircraft-Based Observation data has been shown to be one of the top five most important data sets (measured by impact per observation) for regional and global Numerical Weather Prediction (NWP) models. During the global COVID crisis, the decrease in aircraft-based data reduced global NWP accuracy by more than 20% in some analyses.
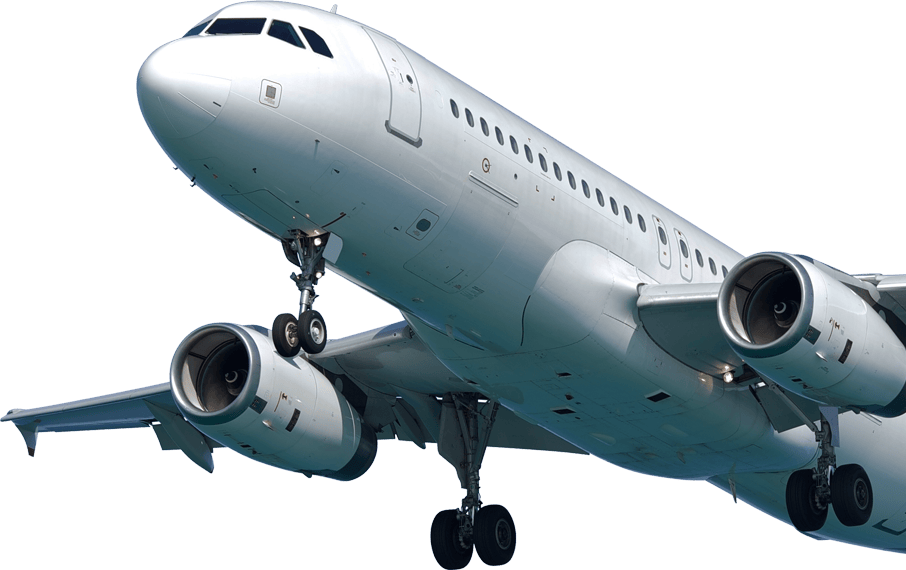
Remote Sensing Technologies like radar and lidar offer additional insights. Radar systems detect precipitation intensity and movement by emitting radio waves that bounce off raindrops, snowflakes, or hailstones. This data is vital for short-term forecasts and severe weather warnings. Lidar and wind profilers provide detailed measurements of wind and atmospheric structures, enhancing the understanding of weather systems.
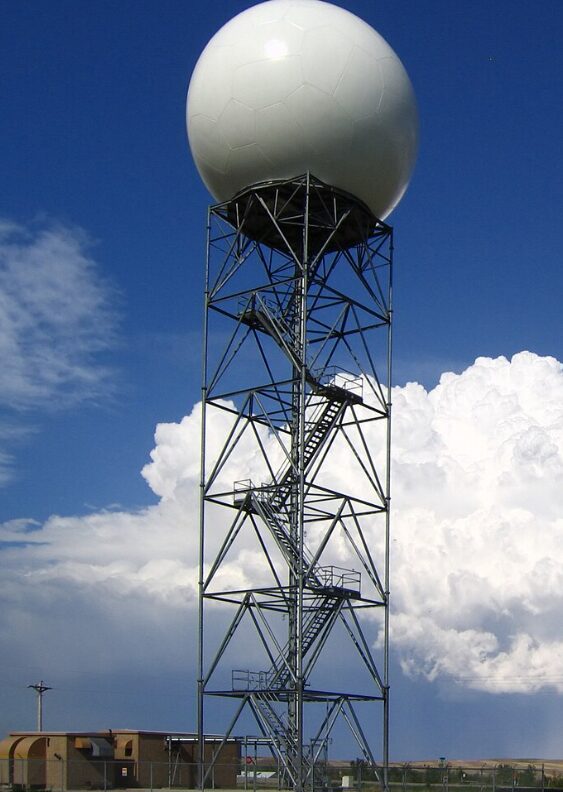
Weather Radar Station
Despite the extensive data collection efforts, challenges remain. Significant data gaps exist over low-population areas and oceans due to the sparse distribution of observation stations. Moreover, ensuring the accuracy and consistency of data from diverse sources is an ongoing task. Quality control procedures are essential to filter out erroneous data that could compromise model accuracy.
International collaboration plays a crucial role in data collection.
Organizations like the World Meteorological Organization (WMO) facilitate the sharing of weather data among countries, creating a global network of observations. This cooperation enhances the overall quality of weather models, as more comprehensive data leads to better initial conditions and, consequently, more accurate forecasts.
1.3 Types of Weather Models
Weather models can be broadly classified into two categories: deterministic models and ensemble models. Each type has its own methodology, strengths, and limitations.
Deterministic Models provide a single, specific forecast based on the best estimate of the current atmospheric state. They use high-resolution grids to simulate weather conditions, capturing detailed features like local temperature variations, wind patterns, and precipitation events. An example is the Global Forecast System (GFS) run by the U.S. National Weather Service, which provides global forecasts with a 13 km resolution for the first ten days.
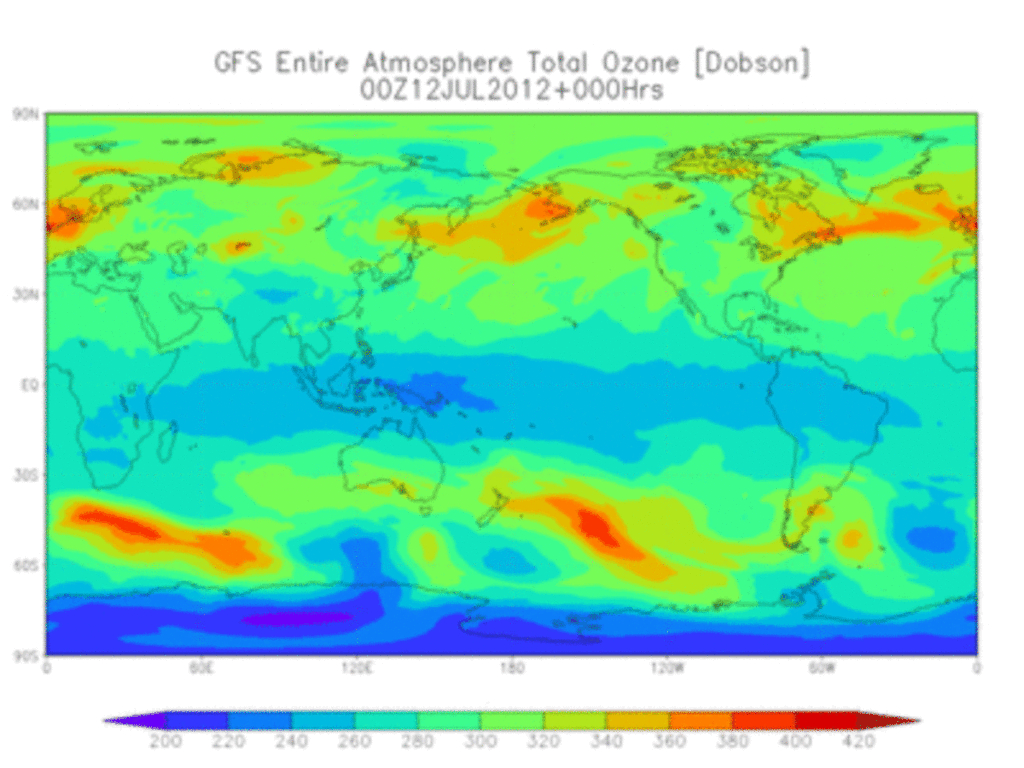
The advantages of deterministic models include their high spatial resolution and operational efficiency. They are capable of delivering detailed forecasts quickly, which is essential for timely decision-making. However, they are sensitive to initial conditions. Small errors in the data used to start the model can amplify over time due to the chaotic nature of the atmosphere, leading to significant deviations in the forecast. Additionally, deterministic models do not provide information about the uncertainty of the forecast, making it challenging to assess the confidence level.
Ensemble Models, on the other hand, generate multiple forecasts by introducing slight variations in initial conditions or model parameters. This approach accounts for the inherent uncertainties in weather prediction. Each individual forecast in the ensemble is known as a member, and collectively, they provide a range of possible outcomes.
The main advantage of ensemble models is their ability to produce probabilistic forecasts. By analyzing the spread of the ensemble members, users can gauge the likelihood of different weather scenarios. This probabilistic information is invaluable for risk management and decision-making under uncertainty. The European Centre for Medium-Range Weather Forecasts (ECMWF) Ensemble Model is renowned for its accuracy and advanced data assimilation techniques, employing four-dimensional variational data assimilation (4D-VAR) for improved forecasts.
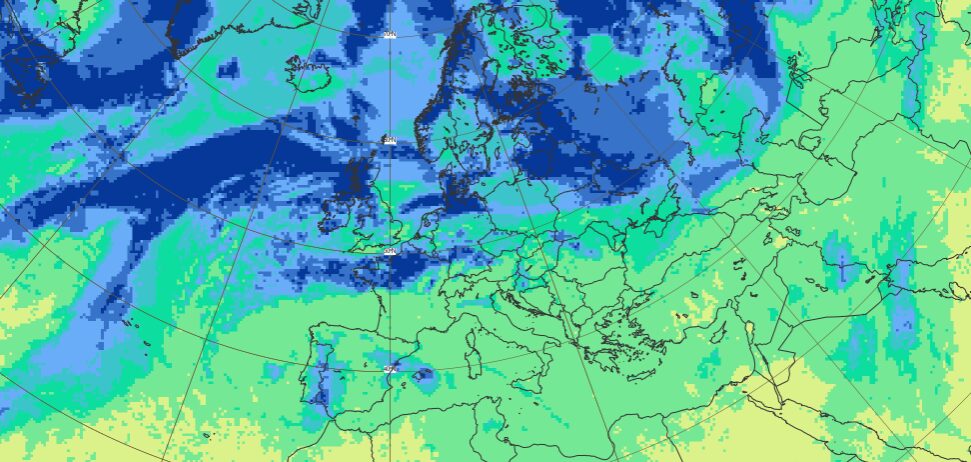
However, ensemble models require significant computational resources due to the need to run multiple simulations. They also produce large amounts of data, which can be complex to interpret without specialized expertise. Despite these challenges, ensemble models are essential for providing a more comprehensive understanding of potential future weather conditions.
Weather models also differ in terms of resolution and grid size. Horizontal grids divide the Earth’s surface into a grid system, where finer grids (higher resolution) capture more detail but demand more computational power. Vertical grids segment the atmosphere into layers to model changes with altitude, crucial for understanding phenomena like temperature inversions and jet streams. Spectral models use mathematical functions to represent atmospheric variables, offering smooth representations but potentially missing localized features.
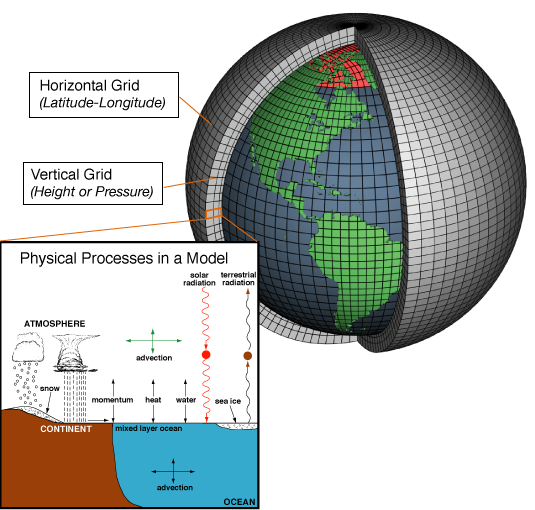
Weather models divide the earth or specific regions into grids, with smaller grids representing higher resolutions
Understanding the types of weather models and their characteristics enables users to select the most appropriate forecasts for their needs. Recognizing the strengths and limitations of each model type is essential for effective interpretation and application of weather data.
1.4 The Canadian Regional Ensemble Prediction System (REPS)
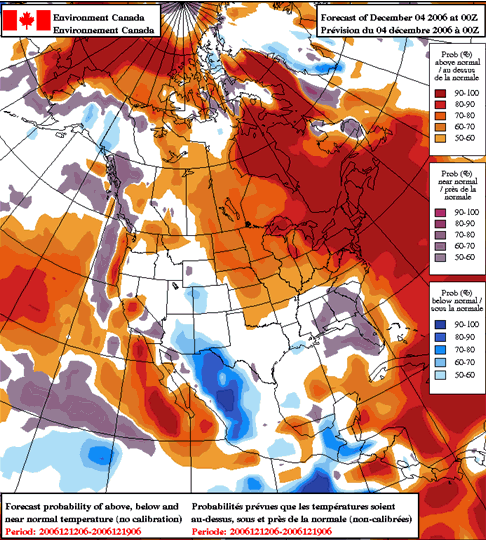
The Canadian Regional Ensemble Prediction System (REPS) exemplifies a high-resolution ensemble model designed to provide detailed short-term weather forecasts for Canada and surrounding regions. By focusing on a specific geographical area, REPS can offer finer grid spacing, capturing localized weather events that might be overlooked by global models.
REPS employs an ensemble approach by running multiple simulations with slight variations in initial conditions and model physics. This method accounts for uncertainties inherent in weather prediction, especially at smaller scales. The probabilistic outputs generated by REPS, such as percentiles and probability maps, help users understand the range and likelihood of possible weather scenarios.
For instance, REPS might indicate a 70% probability of precipitation exceeding 10 mm in a particular region over the next 24 hours. Such information is invaluable for planning and risk assessment, allowing users to make informed decisions based on the likelihood of different outcomes.
The advantages of REPS include its high spatial resolution and frequent updates. The model provides timely information essential for monitoring rapidly changing weather conditions, which is crucial for activities that are sensitive to weather variability.
1.5 Model Initialization and Running
The process of initializing and running weather models is critical to their accuracy and reliability. Initial conditions are established through data assimilation, which integrates observational data into the model’s starting point. This process involves complex algorithms that blend data from various sources, creating a coherent and accurate representation of the current atmospheric state.
Quality control is a vital aspect of data assimilation. Erroneous or inconsistent data can significantly degrade model performance. Statistical methods and physical checks are used to detect and correct anomalies, ensuring that the input data is as accurate as possible.
Weather models are computationally intensive, requiring powerful supercomputers capable of performing trillions of calculations per second. These computers use parallel processing, distributing computations across thousands of processors to handle the large datasets and complex equations efficiently.
To give you an idea of the processing power, you’d need about 20,000 standard laptops to perform the same number of calculations per second as a weather modeling super computer!
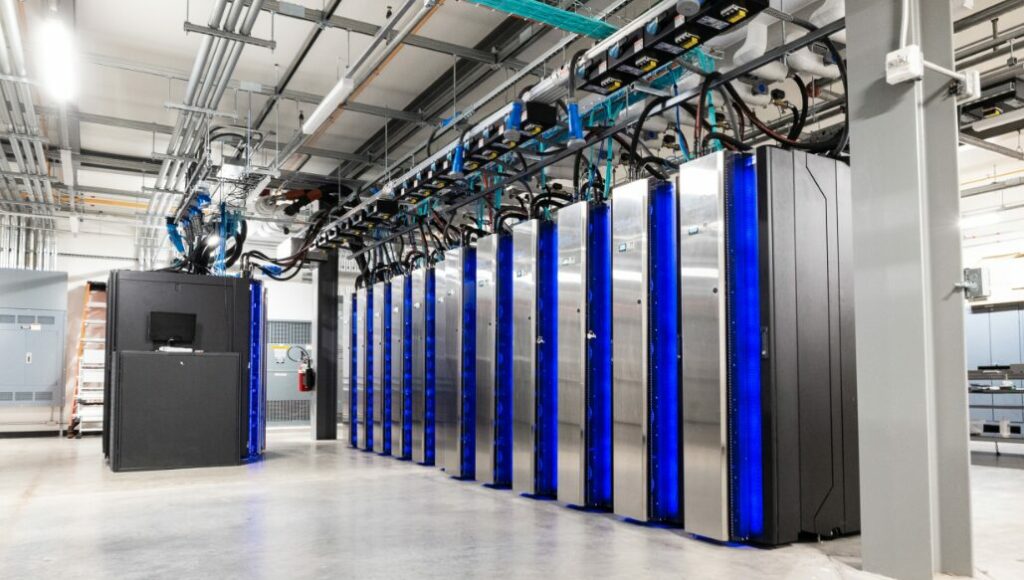
Super computers at NOAA that run weather models
The processing time versus resolution is a critical consideration. Higher resolution models provide more detail but demand more computational resources and time. Therefore, a balance is struck to deliver timely forecasts without compromising essential detail.
Update cycles are another important aspect of model operation. Models are typically updated every six hours (00Z, 06Z, 12Z, 18Z, where Z is Zulu or UTC (universal) time – centered on Greenwich, England) to incorporate new data and capture the latest atmospheric developments. The data collected during these times may vary due to atmospheric conditions, leading to differences in the model outputs. For example, the warm model (00Z) is based on data from the warmer part of the day and may display different characteristics compared to the cold model (12Z), which is based on data from the cooler part of the day.
Model verification involves comparing the model outputs with actual observations to assess accuracy. Consistency over time increases confidence in the model’s reliability. Forecasters play a crucial role in this process, interpreting model data and adjusting forecasts based on known biases or local knowledge. They act as the “forecaster in the loop,” adding value beyond the raw model output.
Understanding how models are initialized and run, as well as their computational requirements and update cycles, provides insight into the factors that influence forecast accuracy. It also highlights the reasons behind variations in different model outputs and the importance of continuous verification and refinement.
Conclusion
Weather models are indispensable tools in modern meteorology, providing the foundation for weather forecasts that impact various aspects of society. By creating mathematical representations of the atmosphere, these models enable us to predict future weather conditions with increasing accuracy.
Understanding the complexity of weather models and the processes involved in their creation enhances one’s ability to interpret forecasts effectively. Recognizing the importance of data collection, the types of models available, and the methods used to initialize and run these models allows users to make informed decisions based on weather data.
Key takeaways from this lesson include:
- Complexity of Models: Weather models are sophisticated systems that simulate atmospheric processes using mathematical equations and vast amounts of data.
- Importance of Data: High-quality, comprehensive data collection is crucial for reliable forecasts. International collaboration enhances data availability and quality.
- Types of Models: Deterministic models provide specific forecasts but lack uncertainty measures, while ensemble models offer probabilistic forecasts that account for uncertainties.
- Model Limitations: All models have inherent limitations due to data gaps, computational constraints, and the chaotic nature of the atmosphere.
- Verification and Interpretation: Continuous model verification and skilled forecasters’ interpretations are essential for improving forecast accuracy and reliability.
By gaining a deeper understanding of weather models, users are better equipped to interpret weather data and apply it effectively in planning and decision-making processes. This knowledge is particularly valuable when working with tools that provide detailed weather information, such as rainfall probabilities and long-range seasonal forecasts, which can have significant implications for various sectors.